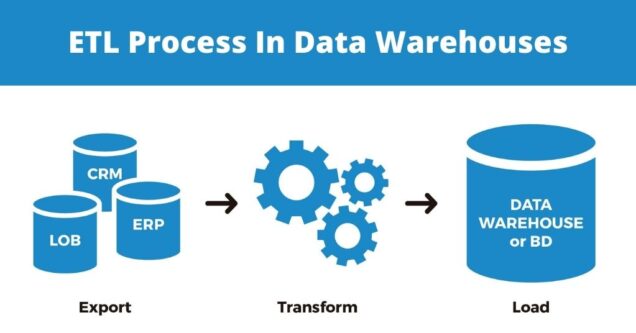
A Detailed Guide Of ETL In Data Warehousing
Table of Contents
Efforts to collect information from external sources (operational systems), alter it to meet business requirements, and finally load the information into a data warehouse are known as enterprise information integration (ETL). Companies utilize extract, transform, and load (ETL) technology to tackle the issue. This technology involves taking data from its source, cleaning it up, formatting it consistently, and then sending it to the destination repository so that it may be exploited.
The current corporate marketplace is heavily reliant on data. With the passage of time, the term “Data” has acquired more relevance since it allows organizations to make choices based on facts and statistics rather than on gut instinct. However, the avalanche of data created on a daily basis limits an organization’s capacity to filter out the noise and get the most relevant information at the most appropriate moment. Businesses will find it challenging to make judgments that will help them become game-changers in their respective industries if they do not have meaningful data at their disposal.
It is at this point that the need for an efficient data warehouse becomes apparent. Managing a data warehouse isn’t as simple as it seems at first glance. When it comes to developing a strong data pipeline, there are several factors to consider, such as which data sources should be used to collect information and how the information should be sent to the warehouse for analysis.
An overview of the ETL process in data warehouses
- You must load your data warehouse on a regular basis in order for it to fulfill its intended function of aiding business analysis. It is necessary to extract and copy data from one or more operational systems into a data warehouse in order to accomplish this task. It is a problem in data warehouse settings to integrate, reorganize, and combine massive amounts of data from a variety of sources across several systems, resulting in a new unified information foundation for business intelligence.
- Data extraction and loading from source systems into a data warehouse are referred to as ETL, which stands for extraction, transformation, and loading and is an acronym for extraction, transformation, and loading. It is important to note that ETL refers to a wide procedure rather than three well-defined processes. Using the abbreviation ETL may be too simple since it omits the transportation step and suggests that each component of the process is unique from the others. In spite of this, the whole process is referred to as ETL.
- The methods and activities associated with ETL Data warehousing have been well-known for many years, and they are not necessarily limited to data warehouse environments: a broad array of proprietary applications and database systems serve as the information technology backbone of every organization. Data must be transferred across applications or systems in order to attempt to integrate them and provide at least two apps with the same image of the outside world. This data exchange was mostly handled by processes that were comparable to what we now refer to as ETL.
How ETL enter cloud computing?
The quantity of data we create and gather is increasing at an enormous rate, and this will remain this way. In addition, we have increasingly sophisticated technologies that allow us to leverage all of our data to generate genuine insights into our company and consumers’ behaviors. It is impossible for typical data warehouse architecture to grow to accommodate and handle that amount of data–at least not at an expense and in a timely fashion.
For all of your data, the cloud is the only location where we can do high-speed, complex analytics and intelligence operations. Data warehouses on the cloud, such as those provided by Amazon Redshift, Snowflake, and Google BigQuery, can scale up and down endlessly to handle nearly any amount of information. The use of massively parallel processing (MPP) in a cloud data warehouse allows for the coordination of large workloads across horizontally scaled clusters of computer resources. On-premises infrastructural facilities purely do not have the speed or scalability that cloud infrastructures do. The cloud has changed the way we manage data, as well as how we create and deploy ETL solutions.
Integrating Data Warehouses in a More Modern Way
When it came to enforcing changes to their data warehouses, many firms depended on out-of-date data integration solutions in the past. However, there is an increasing need for the data warehouse integration process to be made simpler at its core.
An appropriate solution to speed up the ETL Data warehousing and management process is necessary in light of the growing number of challenges that are being encountered. By altering the way data warehouses are integrated, you will also update your organization, decrease repeated modifications, shorten the data copying process, increase agility, and, eventually, lower your total expenditure.